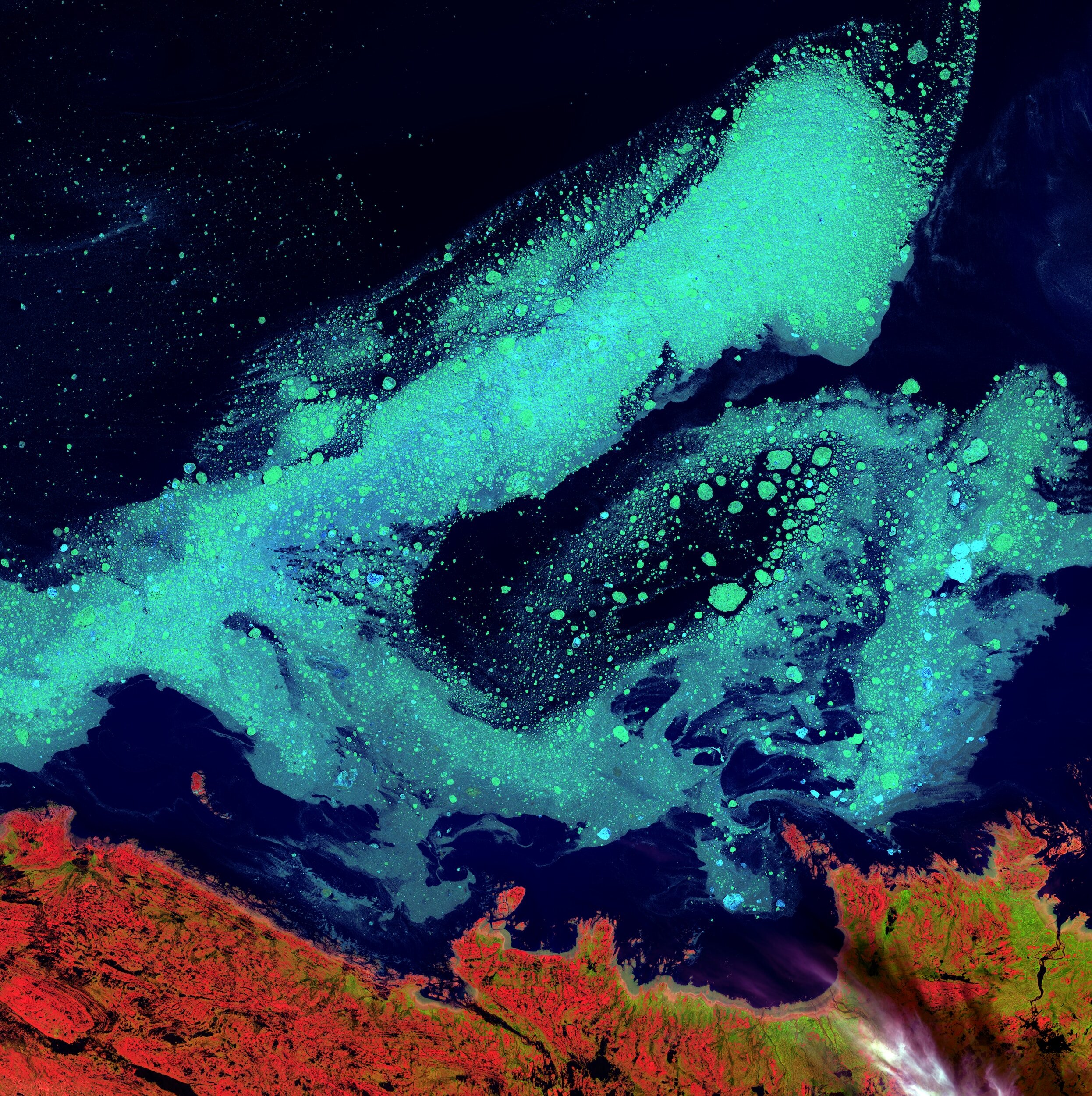
I’d been enthralled by STEM since childhood.
I’d been enthralled by STEM since childhood. So it wasn’t surprising that artificial intelligence captivated me. This deep interest led me to UCSB’s Summer Research Programs for my first experience with research work and first exposure to using machine learning algorithms in the medical field. At UCSB, I researched and coded a GAN model for recreating memories from brain signals. My subsequent paper was published in the “Journal of Student Research” and presented at the UCSB Capstone Seminar. I became obsessed with how AI could be utilized for medical diagnosis, especially for early detection and prevention of illness.
My mom’s arduous cancer recovery inspired me to explore AI’s uses for medical diagnosis and prevention. I’m currently working with Dr. Qing Nie at the NSF-Simon Center to research the performance of different machine learning models in analyzing spatial transcriptomics and scRNA data, which can give doctors a better diagnostic understanding of patients’ bodies.
“I became obsessed with how AI could be utilized for medical diagnosis, especially for early detection and prevention of illness.”
Research Intern, National Science Foundation
Simons Research Center in UC Irvine
2023 - present
Current research intern under Professor Qing Nie for another 1-2 years, researching the application of machine learning in spatial transcriptomics
Incorporating spatial information and gene expression to gain a more comprehensive understanding of a patient’s body that can assist in disease detection
Summer Research Academies in UCSB
June-July 2022
Conducted machine learning research under Dr. Ryan Solgi.
Proposed and designed a GAN (Generative Adversarial Network) model to reconstruct static memories through Electroencephalography
Completed a research paper titled “Reconstructing Static Memories from the Brain With EEG Feature Extraction and Generative Adversarial Networks” and published in the Journal of Student Research, linked here
JOURNAL OF STUDENT RESEARCH
Reconstructing Static Memories from the Brain with EEG Feature Extraction and Generative Adversarial Networks
Forensic tools and facial recognition techniques have implemented state memory reconstruction in the court of law. Recent research has incorporated machine learning into this field in order to fulfill the rising demand for this service. However, the suggested solutions require expensive cutting-edge equipment such as fMRI and CT scanners. The goal of the present study is to recreate images using user electroencephalography (EEG) input patterns. We combined a discriminator and generator network in order to decrease blur, face distortion, and erroneous features in the ImageNet dataset. An EEG feature matrix was produced using a convolutional neural network (CNN) encoder from spectrogram inputs, which are visual representations of the spectrum of electrical frequencies observed at each electrode. The encoder produced an 85% accuracy when linking spectrograms to the labels of the relevant images. The feature matrix was passed into the GAN during training. The viability of image reconstruction and coherent image representation from the brain were both proved feasible to a dependable degree due to our GAN output images’ resemblance to the original dataset. The present work could find applications in future studies as a non-invasive, more affordable option to recreate memories from brain signals.